Artificial Intelligence (AI) has the potential to transform how businesses operate and bring impressive results. Companies that effectively use AI are more likely to see their profits grow. In fact, 44% of business leaders reported a boost in productivity thanks to AI. But there’s a big difference between having data and knowing how to use it.
Imagine a retail company using AI to predict customer preferences. While the data might show a growing demand for eco-friendly products, awareness of this isn't enough. The real magic happens when the company turns this insight into actionable steps—like adjusting inventory, launching targeted marketing campaigns, and training staff. This is what actionable AI is all about.
This article will explore what actionable AI is, its key components, and why it's so important for businesses today. Let’s start our journey.
What is Actionable AI and How Does It Differ from Traditional AI Models?
Traditional AI models are fantastic at processing data and generating insights. They can tell you what's happening or what might happen, but they often stop there. Think of them as insightful advisors—they give you valuable information but don’t always tell you what to do next.
Actionable AI bridges this gap. It’s a smart assistant that gives you great ideas and shows you exactly how to make them happen.
For example, a traditional AI model might identify customer satisfaction dropping because of long wait times. That’s useful information, but actionable AI goes further. It might suggest optimizing staff schedules, implementing a chatbot for quicker responses, or providing targeted training to improve customer service. By focusing on practical applications, actionable AI helps businesses understand their data and act on it effectively.
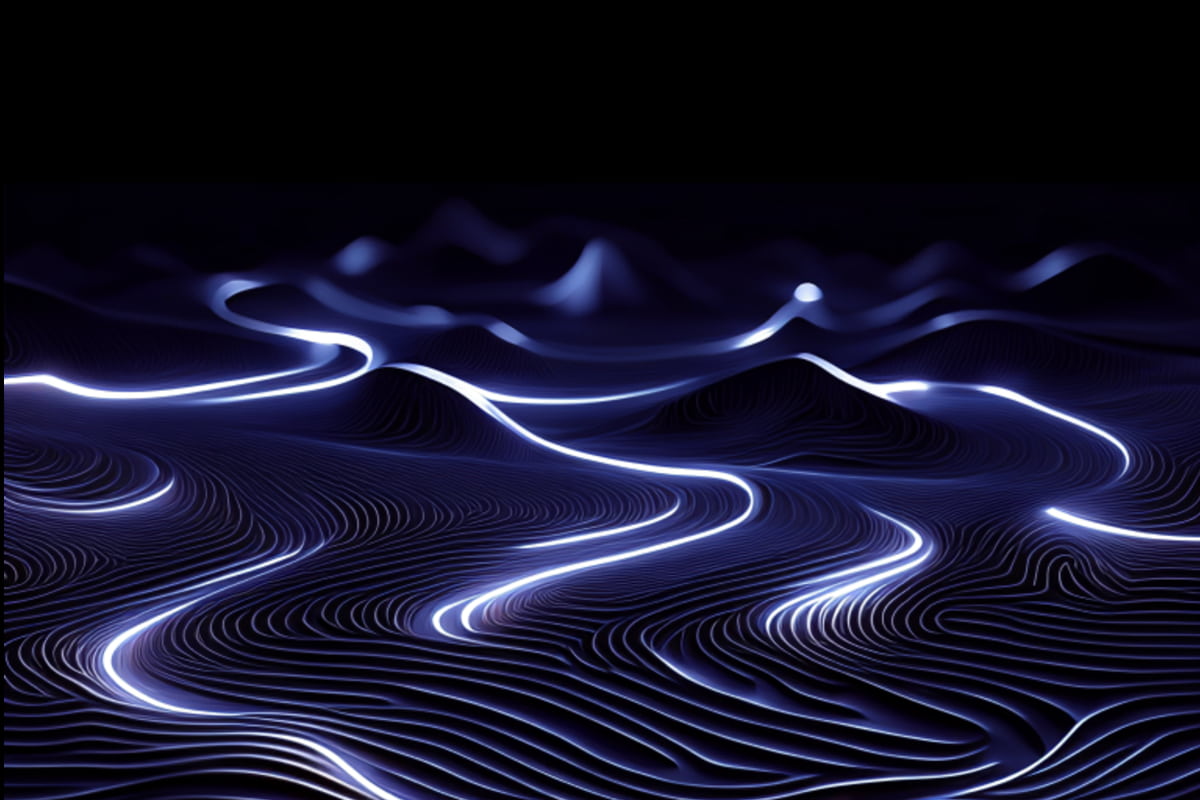
Key Components of Actionable AI
To take full advantage of the power of actionable AI, you should understand its key components: data collection and analysis, insight generation, and implementation mechanisms. Each element is crucial in transforming raw data into practical, impactful actions. Let’s take a closer look at them:
1. Data collection and analysis
Actionable AI starts with data collection and analysis. This involves gathering high-quality data from customer interactions, sales records, and market trends. Technologies like IoT (Internet of Things) devices, web scraping tools, and APIs are commonly used to collect diverse data sources in real-time.
Once the data is collected, analytical tools and techniques such as data mining, statistical analysis, and machine learning help interpret it, identifying patterns and trends. High-quality data is key because the insights and actions AI provides are only as good as the data it's based on. That's why having accurate, relevant, and timely data is so important for making smart decisions.
2. Insight generation
With data in hand, the next step is to generate meaningful insights. This is where machine learning algorithms and data analytics come into play. These models process large datasets to uncover hidden patterns and predict future outcomes. Techniques like clustering, classification, and regression analysis are commonly used to derive insights from data.
For example, a machine learning algorithm might analyze customer purchasing behavior to predict future buying trends. Data analytics tools then help turn these predictions into actionable insights, giving businesses a clear understanding of what the data means and how it can shape their strategies.
3. Implementation mechanisms
The final piece of actionable AI is putting those insights to work in the real world. This means taking the insights generated by AI and applying them to actual scenarios.
Various processes and technologies help make this transition from insight to action smooth and effective, ensuring that businesses can quickly implement AI-driven strategies.
Automation plays a big role here. Automated systems can handle routine tasks based on AI insights, like adjusting inventory levels or personalizing marketing messages. Decision support systems (DSS) are also important, providing managers with the information and recommendations they need to make smart decisions.
A great example is predictive maintenance. In many industries, AI can analyze data from equipment sensors to predict when a machine might fail. It can then suggest preemptive maintenance, which helps reduce downtime and save costs.
Significance of Actionable AI in Modern Business
Now that we’ve covered the key components of actionable AI, let's see why it’s so crucial for modern businesses.
Making data-driven decisions
Actionable AI helps businesses make informed, data-driven decisions. Instead of relying on intuition or outdated information, companies can use actionable AI to analyze current data and generate precise, actionable insights. This leads to more accurate and timely decisions, giving businesses a significant competitive edge.
Enhancing operational efficiency and decision-making processes
Actionable AI not only improves decision-making but also streamlines operations. By automating routine tasks and providing real-time insights, AI enables businesses to operate more efficiently. Here are a few examples:
- Supply chain management: Actionable AI can optimize supply chain processes by predicting demand, managing inventory levels, and identifying potential disruptions before they occur. This reduces waste and ensures products are available when and where they’re needed;
- Customer service: Companies can enhance their customer service operations. For example, AI-powered chatbots can handle common customer inquiries, freeing up human agents to tackle more complex issues. This not only improves efficiency but also enhances the customer experience;
- Predictive maintenance: As mentioned earlier, actionable AI can analyze data from equipment sensors to predict when a machine is likely to fail. By scheduling maintenance before a breakdown occurs, businesses can avoid costly downtime and extend the life of their equipment.
Moreover, actionable AI enhances the speed and accuracy of decision-making processes. For example, decision support systems (DSS) equipped with AI can quickly analyze vast amounts of data, providing managers with real-time recommendations. This allows businesses to react swiftly to market changes, improving overall agility. Sounds great, doesn’t it?
Language-Action Models (LAMs)
Now that we've covered the significance of actionable AI in modern business, let's dive into a specific type of AI model that takes insights to the next level - Language-Action Models (LAMs).
Definition and role of LAMs
Language-Action Models (LAMs) are advanced AI systems designed to understand and generate natural language instructions that can be directly implemented. LAMs are especially handy in situations where human-AI interaction is essential.
Comparison: LLMs vs LAMs
To get a clearer picture of LAMs, let's compare them to another type of AI model: Large Language Models (LLMs). LLMs, like GPT-4, are designed to generate human-like text based on their training data. They excel at producing coherent and contextually relevant text, making them great for tasks like content creation, language translation, and chatbots.
However, while LLMs are fantastic at generating broad insights and text, they often fall short when it comes to translating these insights into specific actions. This is where LAMs shine. LAMs focus on transforming the outputs into action, such as function calling, API request or grabbing an object.
While both LLMs and LAMs leverage natural language processing, their purposes differ. LLMs are great for generating broad insights and text, whereas LAMs are focused on turning these insights into actions. By integrating LAMs into their operations, businesses can benefit from more precise and direct applications of AI-generated insights.
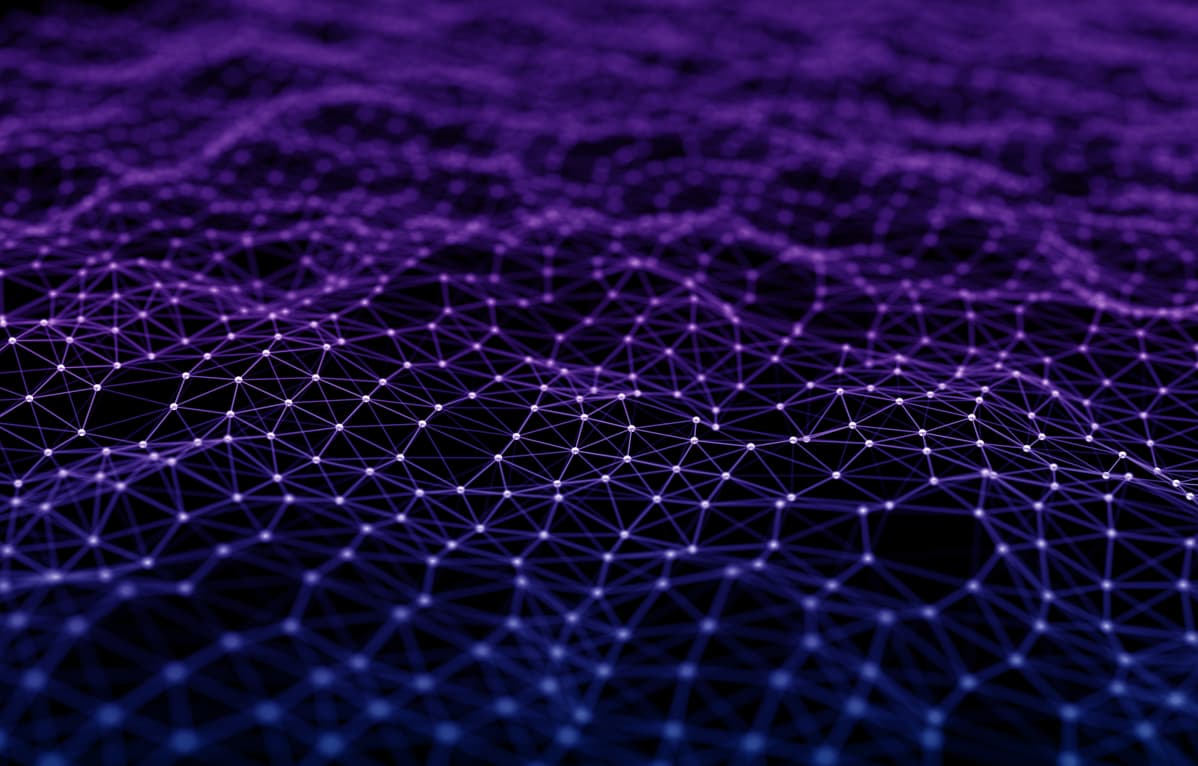
Use Cases of Actionable AI
You are probably wondering how actionable AI is used in the real world. We'll cover strategies for effective implementation and provide examples of companies successfully using actionable AI.
Integrating actionable AI
To successfully integrate actionable AI into your existing business processes, it’s crucial to align AI initiatives with your overall business goals. You should start by identifying key areas where AI can make a significant impact, such as improving customer service, optimizing supply chains, or enhancing decision-making processes.
One effective method we offer is to begin with a pilot project in a specific department or process. This allows you to test the AI system, gather feedback, and make adjustments before scaling it across the organization. Regularly reviewing and aligning AI projects with business objectives ensures that the AI initiatives remain relevant and deliver the desired outcomes.
Ensuring data quality
High-quality data is the foundation of actionable AI. To maintain high data standards, implement best practices for data governance and management. This includes establishing clear data collection protocols, ensuring data accuracy, and maintaining data security and privacy.
Implementing robust data management strategies helps maintain data integrity and makes sure that AI insights are based on accurate and timely information.
Famous companies using actionable AI
- Amazon: Amazon uses actionable AI in various aspects of its operations, from personalized recommendations to supply chain optimization. Their AI algorithms analyze customer behavior and preferences, providing tailored product suggestions that enhance the shopping experience.
- Google: Google leverages actionable AI in its search algorithms and ad targeting. By analyzing user data and search patterns, Google’s AI delivers more relevant search results and targeted ads, driving engagement and revenue.
- Tesla: Tesla uses actionable AI for autonomous driving. Their AI systems analyze data from vehicle sensors to make real-time driving decisions, enhancing safety and efficiency on the road.
- JPMorgan Chase: In finance, AI helps in fraud detection and risk management. JPMorgan Chase uses AI to analyze vast financial transactions and detect suspicious activities in real-time, significantly reducing fraud.
- Zendesk: Zendesk uses actionable AI to enhance customer support. Their AI-driven chatbots handle common customer inquiries, provide instant responses, and escalate more complex issues to human agents, improving both efficiency and customer satisfaction
Future Trends in Actionable AI
As we’ve seen, actionable AI is already making a significant impact across various industries. But what does the future hold for this technology? Let's explore some emerging trends and potential future impacts of actionable AI.
Emerging trends and innovations
- Integration with IoT: One of the most exciting developments is the integration of AI with Internet of Things (IoT) devices. This combination allows for real-time data collection and analysis, helping businesses to make quicker and more informed decisions. Imagine a factory where IoT sensors continuously monitor equipment performance and actionable AI instantly analyzes the data to predict maintenance needs, preventing costly breakdowns.
- NLP development: Another promising trend is the advancement in natural language processing (NLP). As NLP technologies improve, actionable AI systems will become even better at understanding and generating human language. This means more accurate and context-aware interactions, making AI an even more valuable tool in customer service, virtual assistants, and other applications.
- Real-time data processing: Real-time data processing is becoming increasingly important as businesses strive to respond quickly to changing conditions. Actionable AI is evolving to handle massive streams of data in real time, providing immediate insights and recommendations. This capability is crucial in industries like finance, where market conditions can change in an instant, and in healthcare, where timely data can make the difference between life and death.
- AI integration: AI integration is also advancing, making it easier for businesses to incorporate actionable AI into their existing systems. Cloud-based AI platforms are becoming more accessible, offering scalable solutions that can be tailored to specific business needs. This trend allows even small and medium-sized businesses to benefit from advanced AI capabilities.
Potential future impacts
Looking ahead, the opportunities for actionable AI are immense. As AI technologies continue to advance, we can expect even more sophisticated and powerful AI systems that can handle increasingly complex tasks. This will open up new possibilities for innovation and efficiency in every sector.
However, there are also challenges to address. Ensuring data privacy and security is paramount as AI systems become more integrated into our lives. Ethical considerations around AI decision-making and transparency will also need to be carefully managed to maintain trust and fairness.
Conclusion
So, that is a wrap! Actionable AI is bridging the gap between insight and implementation. By turning data into practical steps, companies can make better decisions, streamline operations, and see impressive results. We've explored how actionable AI works, its key components, and its real-world applications.
Ready to take your business to the next level with actionable AI? Feel free to contact us for expert services. Tensorway AI engineers will help you create AI solutions that align with your goals and ensure data quality. Let's use the power of actionable AI to solve your business’ unique challenges.